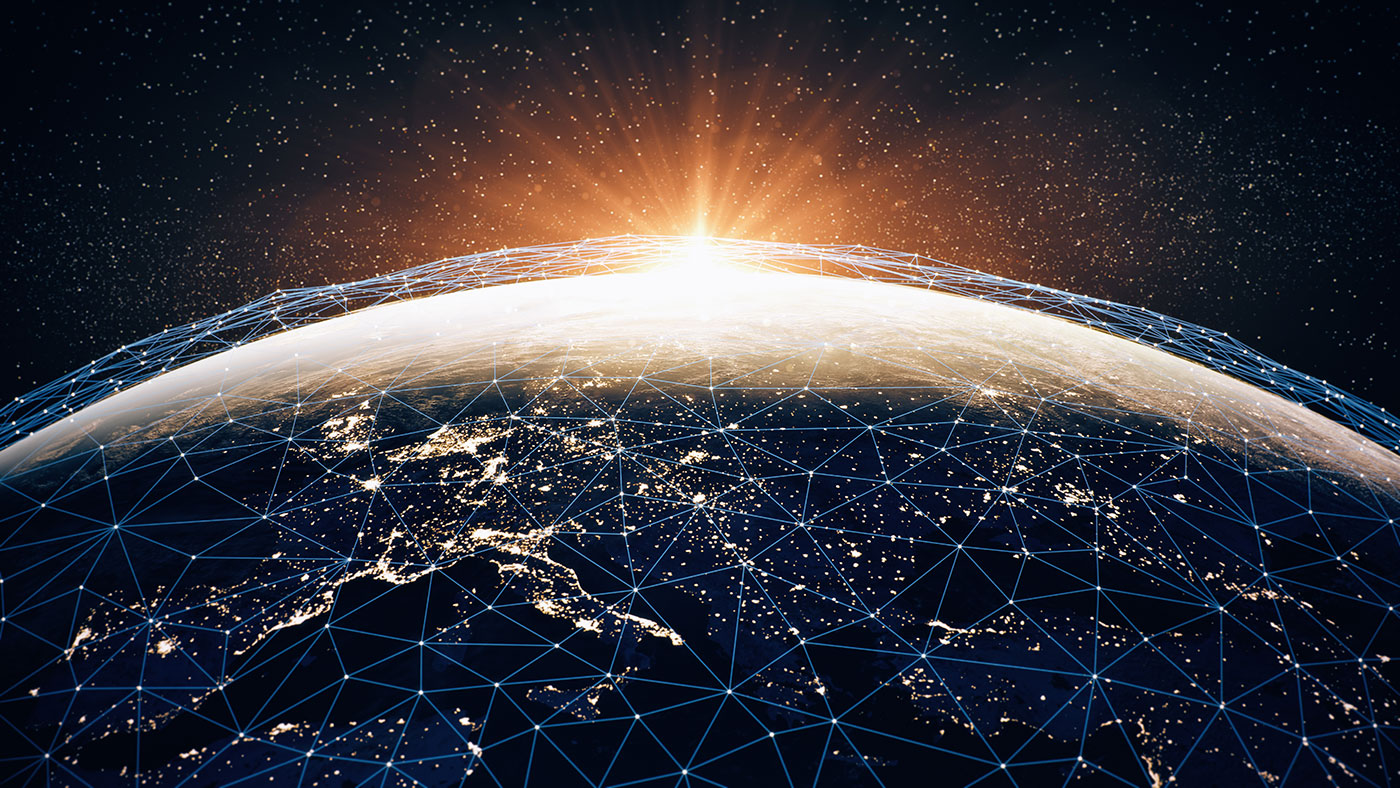
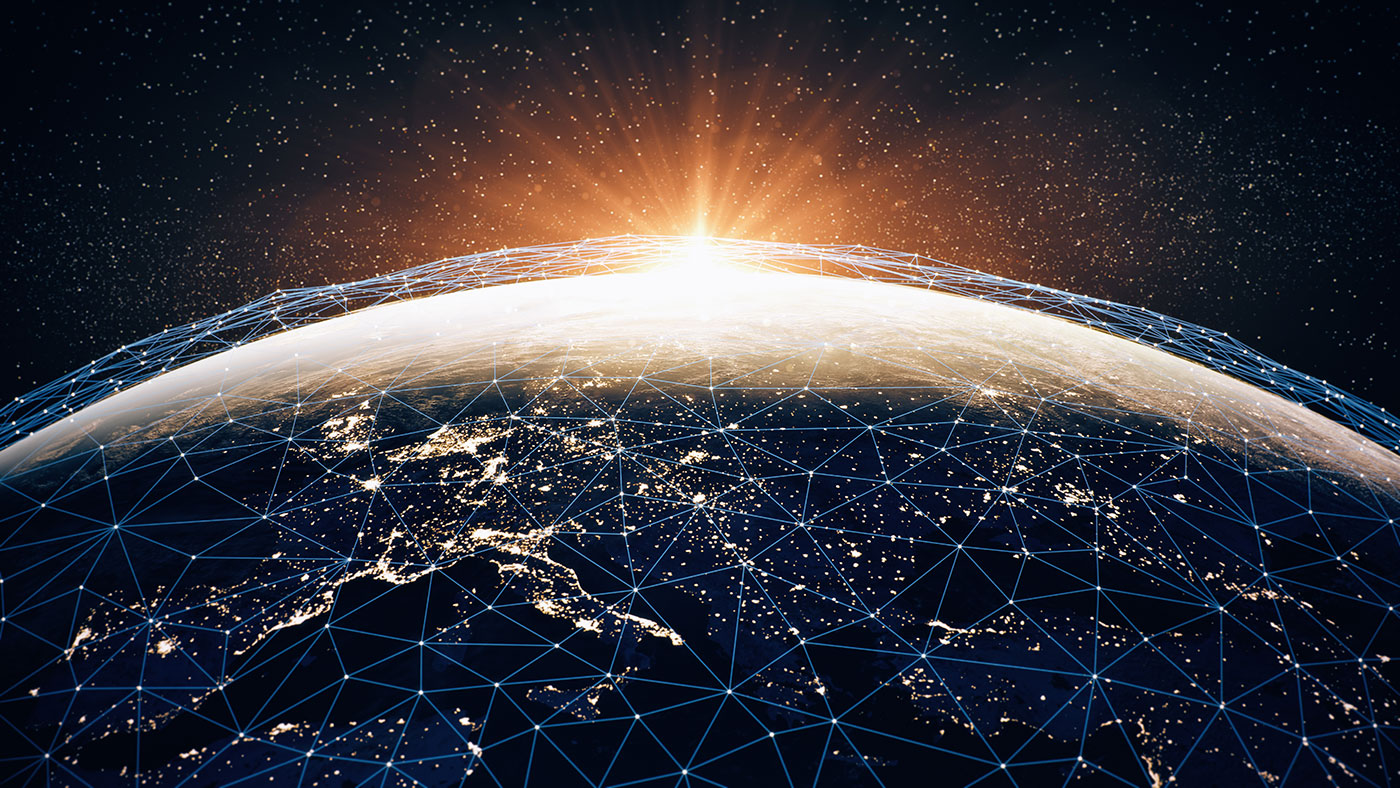
The focus of this thought-piece is the AI dimension of Industry 4.0. This technology area, one of the four grand challenges identified in the UK Industrial Strategy, is a major area of research interest, both from the perspective of the potential for it to be exploited to improve health and safety across UK workplaces, along with the potential for it to introduce new health and safety risks requiring control.
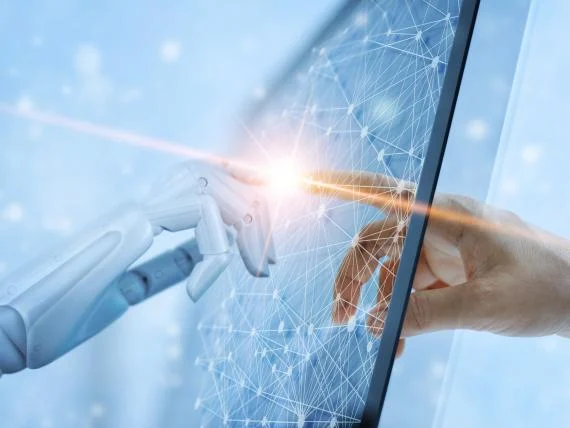
Current State of Play
Examples of industrial use of so called Artificial Narrow Intelligence (ANI) are already numerous across many organisations today. For example, many automated process control systems used across process industries are increasingly using deep learning algorithms as part of the way they operate. In addition, enterprise asset management systems are increasingly making use of Machine Learning (ML) techniques to direct decisions relating to when assets are serviced and checked to maximise up-time and minimise down-time. Examples of more direct use in health and safety contexts are also increasingly commonplace. For example, major hazards industries are starting to use video analytic techniques as part of worksite access control systems, to ensure that workers are using appropriate personal protective equipment when starting work. Use of AI and ML technologies to support health and tasks of a more cognitive nature, for example, the sourcing of advice to ensure regulatory or standards compliance or recognised industry codes of practice are being followed, is also growing across a number of sectors.
Future Opportunities on the Horizon
Such technological exploitation is only likely to grow. For example, many representatives from across asset rich industries, such as aerospace, automotive, power and utilities, are strong advocates of use of AI based technologies to help in the inspection of assets for structural health monitoring purposes to overcome the challenges associated with manual inspection of assets. For assets in remote locations, offshore, for example, or dams and viaducts in rural locations, the safety gains to be realised by combining use of remote visual inspection technologies with AI, ML and video analytic techniques, to negate the need for workers to undertake such tasks, are obviously substantial.
The widespread deployment of CCTV and routine video capture of day to day working practices across workplaces, for example, construction sites and manufacturing spaces, is also opening up opportunities to exploit technologies to auto-detect unsafe working practices and precursors of serious accidents real time. This provides opportunities for health and safety practitioners to intervene early to avoid the more serious accidents happening.
Use of video analytics in combination with AI and ML techniques in this context is also seen by many to offer the potential to significantly improve near miss reporting across organisations. The operation of “near miss” reporting schemes is common place across organisations. The accrual of such intelligence on occurrences that have the potential to lead to much more serious accidents is typically a key element of an organisations health and safety management system, as it helps organisations assess the extent to which prevailing risks are being adequately controlled. However, many near miss reporting schemes are criticised in organisations because of significant under-reporting. The auto-reporting of near misses using CCTV technology in combination with AI and ML driven video analytics offers a potential technology solution to such problems.
The accumulation of vast volumes of data on day-to-day operating experience across workplaces and the ability to link it with a host of individual level data on workers, for example, general health, training and competency records, biometrics, locational data, personal exposures to hazards etc. is providing organisations with the data resource needed to predict the likely occurrence of future adverse health and safety endpoints using statistical machine learning techniques, so called predictive analytics. The availability of such data and use of predictive analytics also offers the potential to tailor risk mitigation measures to individual level circumstances rather than adopting a more “broad brush” approach, so called personalised health and safety, akin to personalised medicine in the clinical domain.
The automation of routine administrative tasks using robotic process automation (RPA) techniques, which typically have AI and ML algorithms at the core, is becoming common place across many organisations. Organisations’ health and safety management systems are no different to other management systems in that key elements are operationalised through a suite of documented administrative procedures. These might include the generation of risk assessments for different work tasks, the identification of specific standards that need to be adhered to when undertaking specific tasks, or the scoping out of methods statements relating to specific work tasks. Many industries, particularly those that are very procedural intensive, are starting to look towards RPA as a way of improving the efficiency with which such administrative tasks are carried out.
It is conceivable that RPA might be taken a step further through the development of AI systems that are able to impart more bespoke health and safety advice. For such systems to be developed, a suitably structured knowledge base would need to be compiled, an inference engine enabling it to be interrogated, along with supporting functionality enabling knowledge requests to be made and knowledge outputs returned. In essence, this describes what is often referred to as an expert system.
Such systems could significantly enhance the way in which regulators share with their duty holders current industry good practice pertaining to how specific health and safety risks might be best mitigated. Equally the systems could provide a way for industry to better ensure that specific work orders are compliant with relevant standards and approved codes of practice.
As technological advances continue, both in AI and ML, and the computing power needed to fuel it, many futurists regard it as inevitable that ANI systems will be replaced by wider or more general intelligence systems, obviously contingent on progress continuing unchecked. The potential, particularly from a cognitive perspective, for AGI systems to change the health and safety landscape across organisations, both from a regulatory and industry perspective, is obviously immense.
Related Content