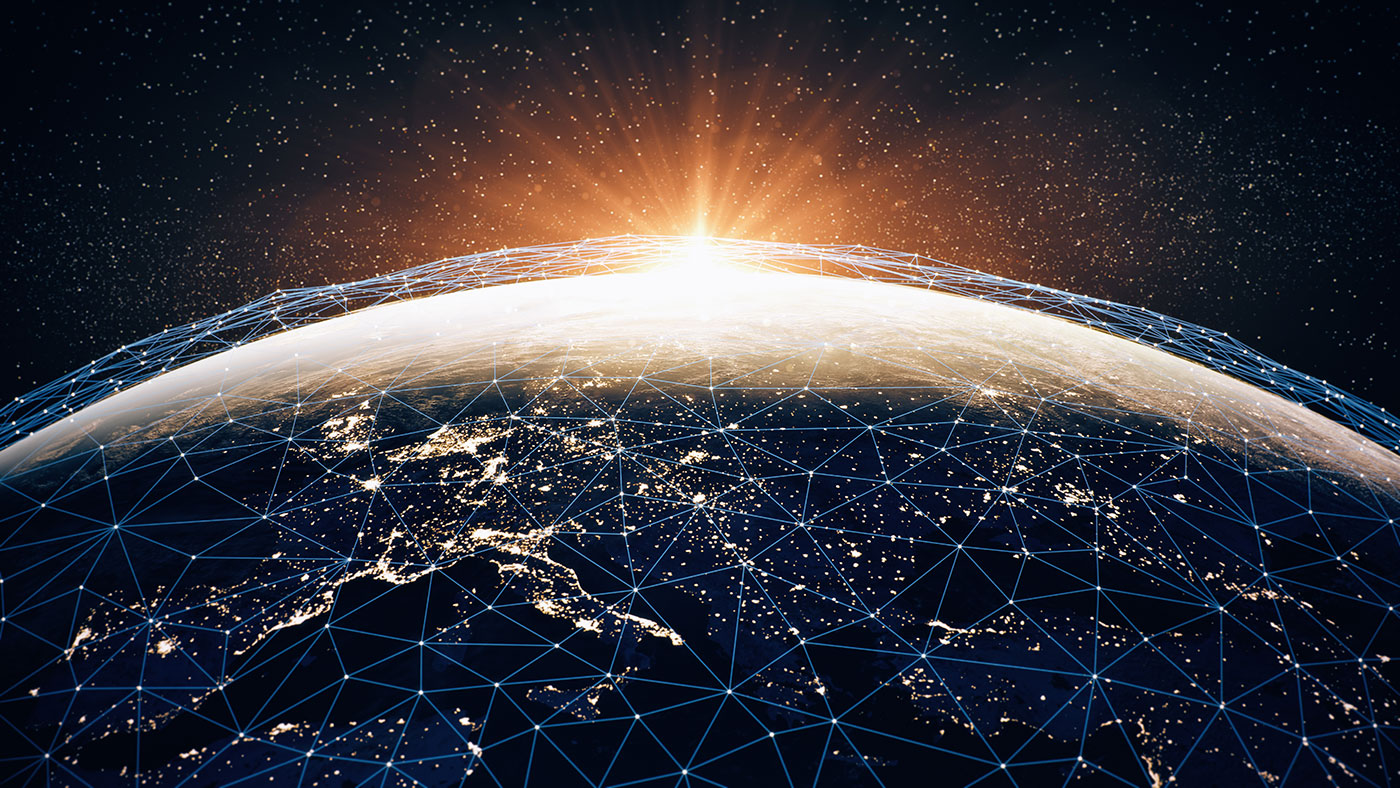
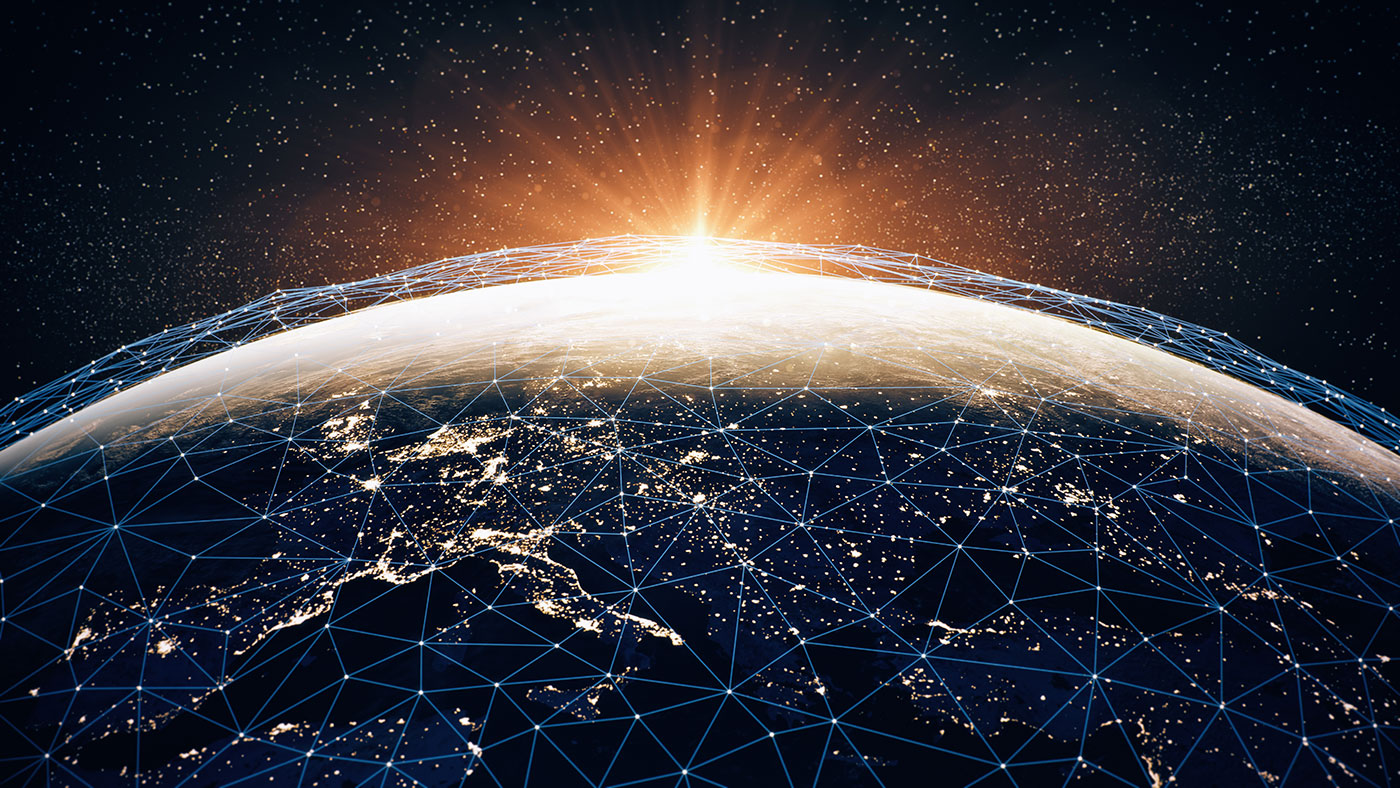
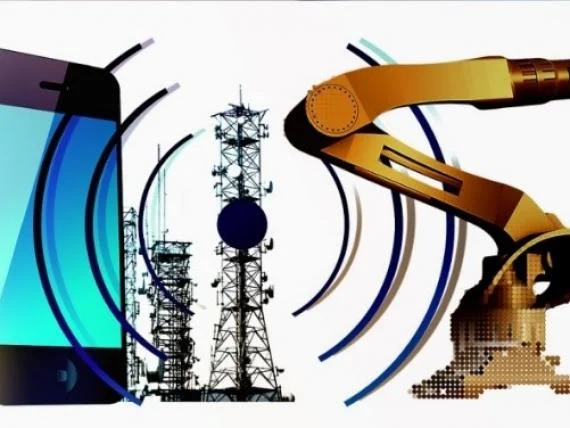
Following on from last weeks blog ‘Rise in industry 4.0 and industrial AI technologies in workplaces and current and future opportunities for more proactive health and safety: Part 1‘, here is part2, third in the series.
In the previous article, I considered the AI dimension of industry 4.0 and offered a perspective on how the emergence in use of industry 4.0 and AI technologies in workplaces is opening up opportunities for more proactive industrial decision-making in relation to how health and safety is practiced. In this article, I consider future technological opportunities on the horizon in a bit more detail.
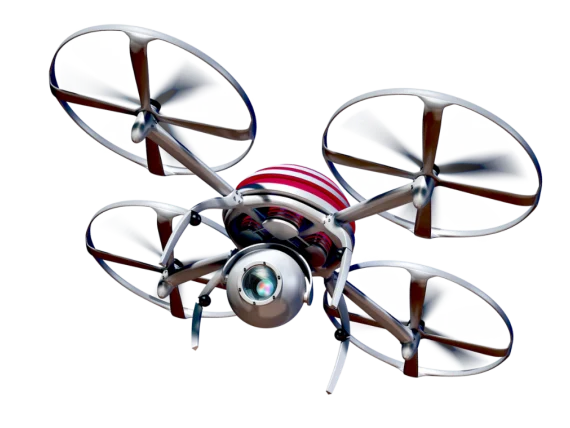
As highlighted previously, real practical examples of deployment of AI based technologies in industrial workplaces, specifically for health and safety purposes, are already numerous and continue to grow. This trend is only likely to continue with many other use cases already on the horizon. For example, many representatives from across asset rich industries, such as aerospace, automotive, power and utilities, are strong advocates of use of AI based technologies to help in the inspection of assets for structural health monitoring purposes, to overcome the challenges associated with manual inspection of assets. For assets in remote locations, offshore, for example, or dams and viaducts in rural locations, the safety gains to be realised by combining use of remote visual inspection technologies with AI, ML and video analytic techniques, to negate the need for workers to undertake such tasks, are obviously substantial.
The widespread deployment of CCTV and routine video capture of day-to-day working practices across workplaces, for example, construction sites and manufacturing spaces, is also opening up opportunities to exploit technologies to auto-detect unsafe working practices and precursors of serious accidents real time. This provides opportunities for health and safety practitioners to intervene early to avoid the more serious accidents happening.
Use of video analytics in combination with AI and ML techniques is also seen by many to offer the potential to significantly improve near miss reporting across organisations. The operation of “near miss” reporting schemes is common place across organisations. The accrual of such intelligence on occurrences that have the potential to lead to much more serious accidents is typically a key element of an organisations health and safety management system, as it helps organisations assess the extent to which prevailing risks are being adequately controlled. However, many near miss reporting schemes are criticised in organisations because of significant under-reporting. The auto-reporting of near misses using CCTV technology in combination with AI and ML driven video analytics offers a potential technology solution to such problems.
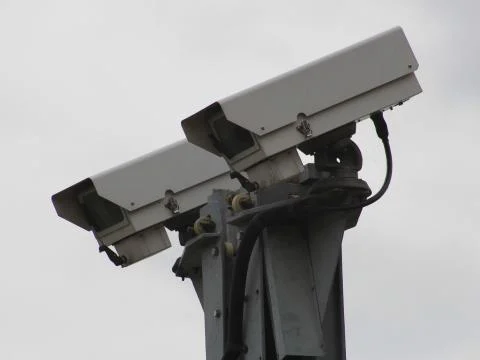
The accumulation of vast volumes of data on day-to-day operating experience across workplaces and the ability to link it with a host of individual level data on workers, for example, general health, training and competency records, biometrics, locational data, personal exposures to hazards etc. is providing organisations with the data resource needed to predict the likely occurrence of future adverse health and safety endpoints using statistical machine learning techniques, so called predictive analytics. The availability of such data and use of predictive analytics also offers the potential to tailor risk mitigation measures to individual level circumstances rather than adopting a more “broad brush” approach, so called personalised health and safety, akin to personalised medicine in the clinical domain.
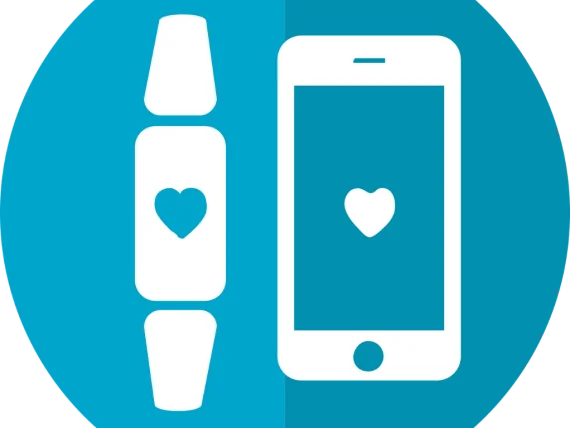
The automation of routine administrative tasks using robotic process automation (RPA) techniques, which typically have AI and ML algorithms at the core, is becoming common place across many organisations. Organisations’ health and safety management systems are no different to other management systems in that key elements are operationalised through a suite of documented administrative procedures. These might include the generation of risk assessments for different work tasks, the identification of specific standards that need to be adhered to when undertaking specific tasks, or the scoping out of methods statements relating to specific work tasks. Many industries, particularly those that are very procedural intensive, are starting to look towards RPA as a way of improving the efficiency with which such administrative tasks are carried out.
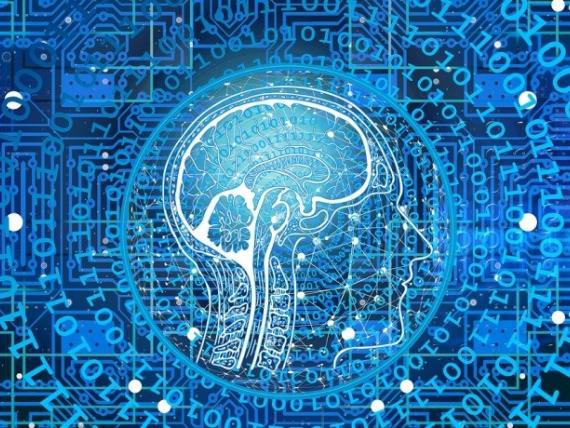
It is conceivable that RPA might be taken a step further through the development of AI systems that are able to impart more bespoke health and safety advice. For such systems to be developed, a suitably structured knowledge base would need to be compiled, an inference engine enabling it to be interrogated, along with supporting functionality enabling knowledge requests to be made and knowledge outputs returned. In essence, this describes what is often referred to as an expert system. Such systems could significantly enhance the way in which regulators share with their dutyholders current industry good practice pertaining to how specific health and safety risks might be best mitigated. Equally the systems could provide a way for industry to better ensure that specific work orders are compliant with relevant standards and approved codes of practice.
A final area of AI application that is garnering significant R&D interest currently is in the development of artificially intelligent robots and cobots. Currently, robots and cobots tend to be explicitly programmed to undertake repetitive tasks, for example, routine physical tasks on assembly lines. However, many feel it is inevitable that in time such technologies will be replaced with equivalents under the control of machine and deep learning algorithms. This will enable them to undertake much more diverse tasks across workplaces.
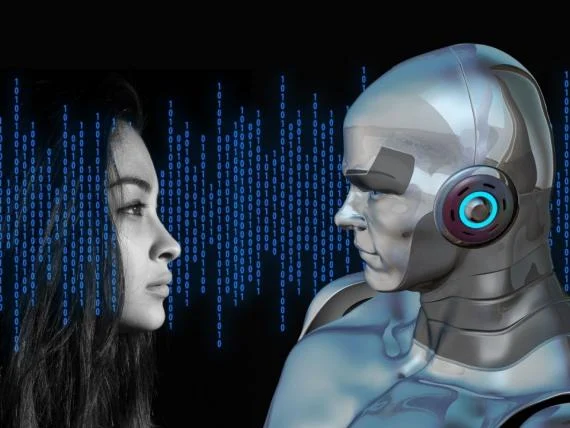
As technological advances in AI continue and the computing power needed to fuel it, many futurists regard it as inevitable that current systems will be replaced by more complex systems. However, one of the implications of AI based technologies being used to deliver more diverse, complex tasks across workplaces is that there are more opportunities for such tasks to be risky from a health and safety perspective. As the risk landscape becomes more diverse and complex, it inevitably becomes much more challenging to manage and regulate. These areas will provide the focus in a future article.
Look out later this week for my forth blog in this series ‘Rise in industry use of AI technologies in workplaces and future challenges for health and safety: Part 1’ which considers some of the challenges faced in deploying technologies effectively.
Related Content